What Are the Impacts of Machine Learning On Fintech Products?
Fintech Interviews

What Are the Impacts of Machine Learning On Fintech Products?
In the rapidly evolving world of financial technology, machine learning is making waves with its transformative impact. We've gathered insights from top fintech professionals, including data scientists and product managers, to share specific instances where machine learning has revolutionized their products. From detecting fraudulent insurance claims to improving invoice payment clearance, here are four compelling examples of machine learning in action.
- Detecting Fraudulent Insurance Claims
- Revolutionizing Credit Scoring Accuracy
- Automating Document Processing with ML
- Improving Invoice Payment Clearance
Detecting Fraudulent Insurance Claims
Machine Learning (ML) had a significant impact on the finance industry where I worked, specifically in the detection of bogus insurance claims. In the Fintech industry, insurance services were provided, including claims processing for various forms of insurance (such as health, car, and property).
One of the most challenging tasks we faced was recognizing and blocking false insurance claims, which could result in substantial financial losses for the organization. ML can detect and minimize fraudulent activity as it occurs. It can also predict future behavior and recommend prevention strategies.
Forensic analysis can investigate the causes of a fraud incident and the relationships between the various elements that contributed to it. Implementing an ML model tailored exclusively for detecting fraud in insurance claims, the model incorporated a variety of data points and attributes from each claim, including Claim Details, User Behavior, and External Data. It analyzed the type of claim (e.g., accident, theft), claim amount, policy details, and the insured party's history. It investigated the patterns in which claims were submitted, the frequency of claims, and any unusual changes in behavior. External sources, such as historical data on similar claims, public records, and social media data (where applicable and legally acceptable), were used to validate claims and analyze them.
The ML model adjusted its detection parameters dynamically based on past data, resulting in fewer false alarms than traditional rule-based systems, which frequently produced false positives. Incorporating machine learning into our insurance claim fraud detection system not only strengthened our defenses against monetary losses but also expedited processes and increased client satisfaction.
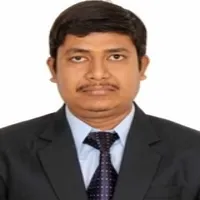
Revolutionizing Credit Scoring Accuracy
At Miquido, we've partnered with Nextbank, a fintech company providing banking software to leading Asian banks, to develop one of the first ML-powered credit scoring systems.
Traditional credit scoring methods fell short for Nextbank, being costly, time-consuming, and slow to adapt to economic changes, often resulting in biased lending decisions.
By integrating advanced ML models like LightGBM and XGBoost, we revolutionized Nextbank’s credit scoring process. These models analyzed over 600 data points from demographics, transaction histories, and credit records to enhance credit score accuracy and provide a deeper understanding of credit risk.
The impact was profound. The new ML-powered system achieved a 97% accuracy rate, processed over 500 million loan applications, and significantly reduced default risks. The system continuously improved by learning from new data, ensuring lending decisions were based on the most current and comprehensive information. This led to better risk management and a reduction in bias, as the models relied on actual repayment data rather than human judgment.
This project not only optimized lending decisions for Nextbank but also showcased how machine learning can modernize the financial services industry, making it more efficient, accurate, and fair.
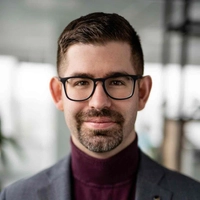
Automating Document Processing with ML
In the world of Intelligent Document Processing, we specialize in serving clients in the financial services industry. With the increasing volume of documents and many clients requesting faster turnaround times, we found ourselves overwhelmed by the demands and constrained by our resources.
To address this, we turned to the growing wealth of Machine Learning technologies, including Large Language Models (LLMs) and Geospatial Layout Models. By effectively utilizing these models, we managed to automate approximately 80% of the document processing with 99% accuracy. This significantly improved our client retention, opening new growth opportunities and increasing customer satisfaction.
From my experience, I advise that while leveraging the latest machine learning technology, it's essential to recognize that human intelligence guides artificial intelligence. When tackling a machine learning problem, breaking it down into distinct parts and training models accordingly for each piece can help mitigate issues like overfitting and data pollution, and give a higher prediction accuracy overall.
Improving Invoice Payment Clearance
FinTech products come in a variety of flavors, one of which is Invoice Payments and Clearance. Partners transferring money to us via the Bank Transfer Payment Method often fail to mention the invoice reference, or this reference gets lost in translation when their bank settles money in our bank. We at Booking have enabled an ML product called 'Smart Clearance,' which matches the amount, date, transaction reference, and other parameters with the invoice and billing cycle to appropriately assign the payment to the partner and clear their outstanding invoices, thus avoiding open balance aging, bad debt, and unnecessary partner suspensions. We improved our auto-clearance rate from 78% to 89%, and more enhancements are underway to achieve a 99.9% clearance rate goal.
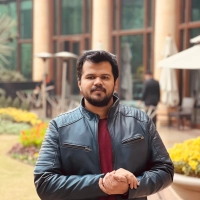